MPIIGroupInteraction
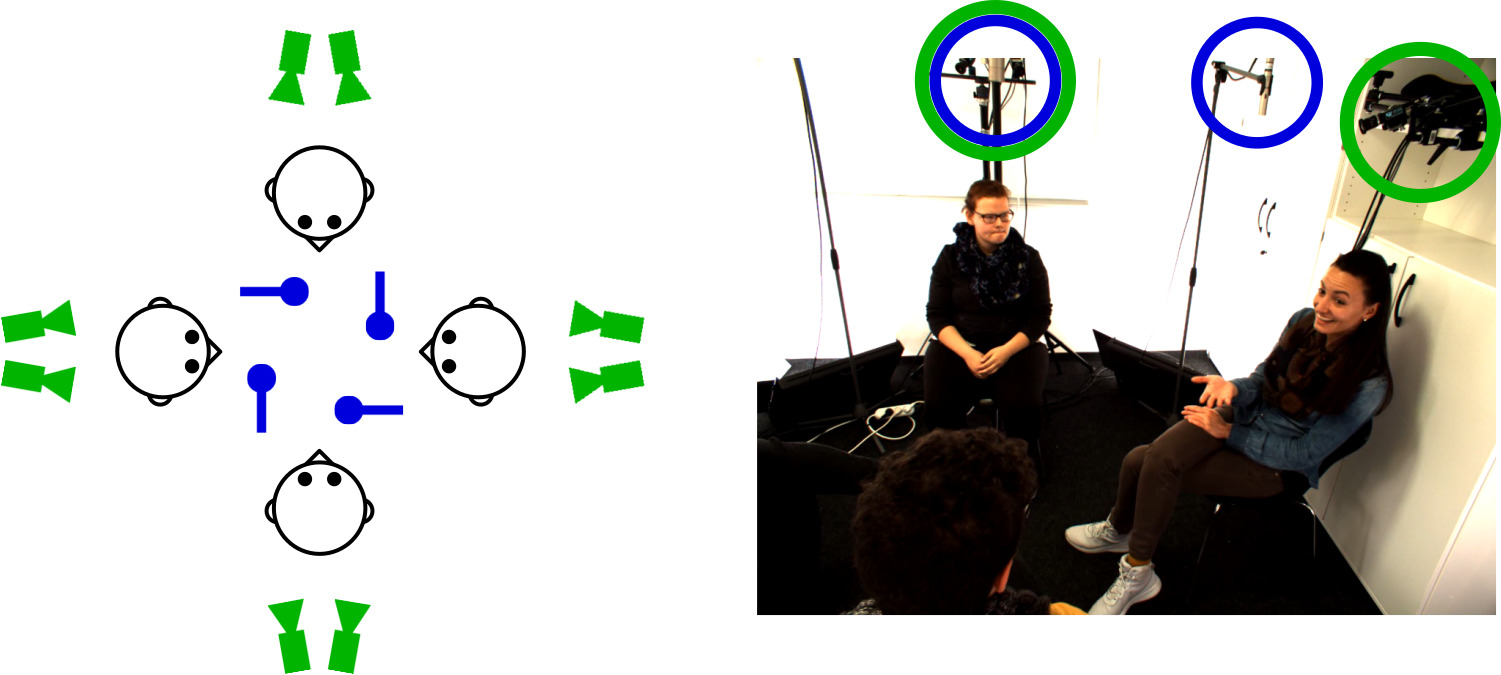
Recording setup
The data recording took place in a quiet office in which a larger area was cleared of existing furniture. The office was not used by anybody else during the recordings. To capture rich visual information and allow for natural bodily expressions, we used a 4DV camera system to record frame-synchronised video from eight ambient cameras. Specifically, two cameras were placed behind each participant and with a position slightly higher than the head of the participant (see the green indicators in the figure). With this configuration a near-frontal view of the face of each participant could be captured throughout the experiment, even if participants turned their head while interacting with each other. In addition, we used four BehringerB5 microphones with omnidirectional capsules for recording audio. To record high-quality audio data and avoid occlusion of the faces, we placed the microphones in front of but slightly above participants (see the blue indicators in the figure above).
Recording Procedure
We recruited 78 German-speaking participants (43 female, aged between 18 and 38 years) from a German university campus, resulting in 12 group interactions with four participants, and 10 interactions with three participants. During the group forming process, we ensured that participants in the same group did not know each other prior to the study. To prevent learning effects, every participant took part in only one interaction. Preceding each group interaction, we told the participants that first personal encounters could result in various artifacts that we were not interested in. As a result, we would first do a pilot discussion for them to get to know each other, followed by the actual recording. We intentionally misled the participant to believe that the recording system would be turned on only after the pilot discussion, so that they would behave naturally. In fact, however, the recording system was running from the beginning and there was no follow-up recording. To increase engagement, we prepared a list of potential discussion topics and asked each group to choose the topic that was most controversial among group members. Afterwards, the experimenter left the room and came back about 20 minutes later to end the discussion. Finally, participants were debriefed, in particular about the deceit, and gave free and informed consent to their data being used and published for research purposes.
Annotations
* Bodily Behaviours: We make use of 14 behaviour classes from the BBSI annotations. Details on the original annotation procedure can be found in (Balazia et al., 2022). Based on the BBSI annotations, we sampled 64-frame snippets. This is a multi-label task, i.e. several of the 14 classes can be present at the same time. Participants need to predict a score for each sample and class which will be evaluated using average precision.
* Backchannels: Annotators were asked to label the occurrences of backchanneling behaviour on the full dataset. Instead of using a lexicon of utterances (e.g. "a-ha", "yes",...) or nonverbal behaviours (e.g. nodding) that indicate a backchannel, annotators relied on their holistic perception of whether a backchannel occurred or not. The resulting backchannels may be expressed verbally, para-verbally, non-verbally, or with a combination of those modalites. For the backchannel detection task, we created positive and negative examples in the following way. Positive samples (i.e. backchannel samples) were created by aligning the end of the 10 second input window with the end of the backchannel. Negative samples (i.e. no backchannel samples) were created by randomly sampling 10 second windows with the constraint that no backchannel occurs in the last second of the window.
* Agreement expressed in backchannels: Three independent raters annotated each backchannel with the amount of agreement it expresses towards the current speaker. The annotation is dependent on the context. For example, a negative affect display might indicate agreement with the speaker if it validates what the speaker is saying. To obtain agreement ground truth, we average the ratings of all annotators. For the agreement estimation task, we use the positive samples from the backchannel detection task. The end of the backchannel for which the agreement has to be predicted coincides with the end of the 10 second input window.
* Eye contact: 6254 frames on the dataset were annotated with eye contact information. These annotations indicate whether a participant is looking at another participant’s face at a given moment in time, and if yes, who this other participant is.
* Speaking status: We annotated all recordings with respect to the current speaker according to a strict annotation protocol. Besides speech during longer utterances, the annotators were instructed to label back-channels (e.g. "mhm" or "right") and short affirmative or dissenting statements (e.g "yes", "no") as speaking. Nonverbal sounds like coughing or laughing were explicitly labelled as not speaking, as were longer pauses during an utterance that noticeably impact the flow of speech (e.g. thinking pauses). In cases where it was still difficult to assess if a person is speaking, for example if the voice of the speaker is very quiet or sounds similar to that of another speaker, annotators were encouraged to take body language and lip movements into account.
Challenge Dataset
For bodily behaviour recognition, we provide frontal- as well as two side views on each participants. For eye contact estimation, next speaker prediction, backchannel detection, and agreement estimation, we will provide a version of the MPIIGroupInteraction dataset that contains a single frontal view on each participant as well as audio recorded from a single microphone per interaction.
Download: Please download the EULA here and send to the address below. We will then give you the link to access the dataset, annotations and readme.
Contact: Anna Penzkofer, anna.penzkofer@vis.uni-stuttgart.de
The data is only to be used for non-commercial scientific purposes. If you use this dataset in a scientific publication, please cite the following papers:
-
Bodily Behaviors in Social Interaction: Novel Annotations and State-of-the-Art Evaluation
Proceedings of the 30th ACM International Conference on Multimedia, pp. 70–79, 2022.
-
MultiMediate: Multi-modal Group Behaviour Analysis for Artificial Mediation
Proc. ACM Multimedia (MM), pp. 4878–4882, 2021.
-
Detecting Low Rapport During Natural Interactions in Small Groups from Non-Verbal Behavior
Proc. ACM International Conference on Intelligent User Interfaces (IUI), pp. 153-164, 2018.
-
Robust Eye Contact Detection in Natural Multi-Person Interactions Using Gaze and Speaking Behaviour
Proc. ACM International Symposium on Eye Tracking Research and Applications (ETRA), pp. 31:1-31:10, 2018.